
In this paper we would like to present (i) a fast and generic method to evaluate chemical diversity, (ii) a new quantum chemical dataset of 435k molecules, OD9, that includes QM9 and new molecules generated with a diversity objective, (iii) an analysis of the diversity impact on unconstrained and goal-directed molecular generation on the example of QED optimization. As a consequence, ML models trained on QM9 showed generalizability shortcomings. Previously we have seen that the most known quantum chemical dataset QM9 lacks chemical diversity. The composition of which should be done meticulously since the calculation is highly time demanding. This is particularly true for quantum chemical datasets. Follow-up applications include analog searching and core structure-property relationships analyses.Ĭhemical diversity is one of the key term when dealing with machine learning and molecular generation.
MOLECULAR SCAFFOLD MEANING CODE
The code to use the method presented in this work is freely available as an additional file. New statistical methods are envisioned that will be able to draw quantitative conclusions from these data. The molecule-core network herein presented is a general methodology with multiple applications in scaffold analysis.
MOLECULAR SCAFFOLD MEANING SERIES
The applications range from inter-and intra-core diversity analysis of compound data sets, structure-property relationships, and identification of analog series and ASBS. We present case studies illustrating the potential of the general framework. Compounds linked to the same cores are considered analogs. Thereafter, a bipartite network consisting of molecules and cores can be constructed for a database of chemical structures. A putative core is here defined as any substructure of a molecule complying with two basic rules: (a) the size of the core is a significant proportion of the whole molecule size and (b) the substructure can be reached from the original molecule through a succession of retrosynthesis rules. As an extension to ASBS, we herein introduce the development of a general conceptual framework that considers all putative cores of molecules in a compound data set, thus softening the often applied "single molecule-single scaffold" correspondence. In this context, analog series-based scaffolds (ASBS) are synthetically relevant core structures that represent individual series of analogs. Scaffold analysis of compound data sets has reemerged as a chemically interpretable alternative to machine learning for chemical space and structure-activity relationships analysis.
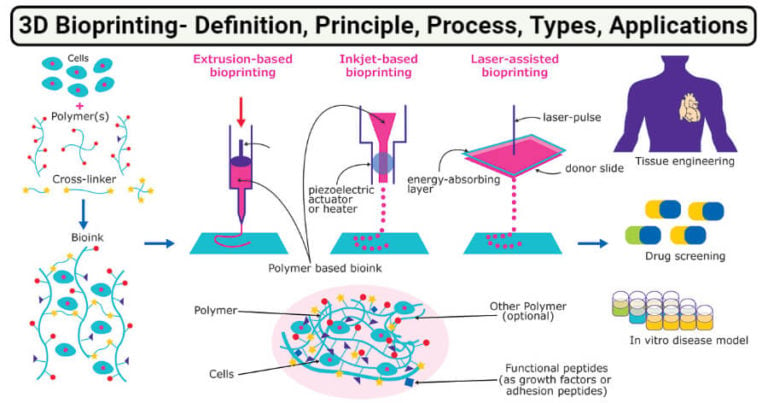
A number of scaffolds of varying chemical complexity have also been detected to form multi-target activity cliffs across different families. The concept of privileged substructures (PSS) was originally introduced in 1988 by Evans and co-researchers, who recognized that the benzodiazepine framework was contained in many ligands of different G-protein coupled receptors (GPCR) and ion channels. In their original scaffold study, Bemis and Murcko analyzed a set of 5000 drugs and found that 25% of these drugs were represented by the 42 most frequently occurring BM scaffolds and 50% by the 32 most frequent cyclic skeletons (CSK), which indicated that the diversity of drug shapes was rather limited. In 1996, a seminal article by Bemis and Murcko introduced a hierarchical molecular organization scheme by dividing small molecules into R-groups, linkers, and frameworks. Some of the studies that have analyzed scaffold distributions in selected compound data sets or in currently available bioactive compounds are reported.
